Exploring Quantitative Methods in Finance
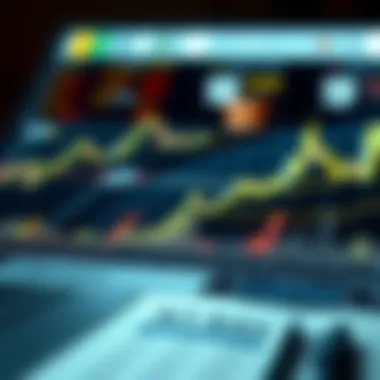
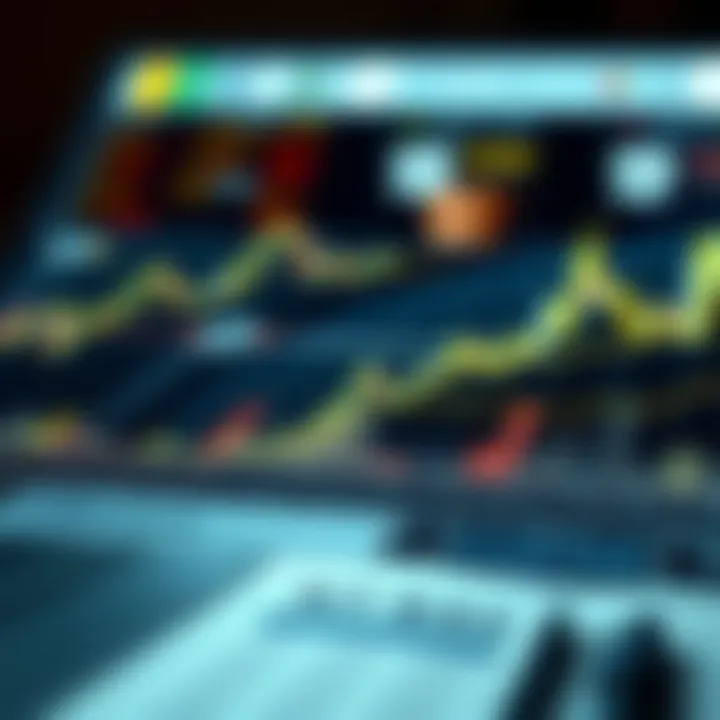
Intro
Exploring the world of finance can often feel akin to wandering through a labyrinth. Amidst the complex instruments and strategies, one area is particularly crucialโquantitative methods. These are not just fancy calculations; they are lifelines for todayโs investors, decision-makers, and financial advisers. Understanding how to apply these methods can mean the difference between navigating turbulent market waters and capsizing in the sea of financial insecurity.
Quantitative analysis allows financial professionals to sift through vast amounts of data, drawing insightful conclusions that can significantly shape investment strategies. But what exactly do we mean by 'quantitative methods'? In simpler terms, itโs the application of mathematical and statistical techniques to analyze financial data. This approach facilitates decision-making by providing a clear, data-driven lens through which investments can be evaluated.
As we navigate through this article, we will peel back the layers and dive into essential definitions, investment product glossaries and much more, arming you with the knowledge to enhance your financial acumen.
Essential Definitions
Overview of Key Investment Terms
In the realm of finance, using the right terminology is crucial. Here are a few key terms you will encounter throughout the discussion on quantitative methods:
- Algorithmic Trading: This technique employs algorithms to determine trading strategies, executing orders with minimal human intervention.
- Risk Management: The practice of identifying, assessing, and prioritizing risks, followed by coordinated efforts of resources to minimize, monitor, and control the impact of unfortunate events.
- Portfolio Diversification: This is the process of spreading investments across various financial instruments, industries, and other categories to reduce risk.
- Quantitative Easing: An unconventional monetary policy used by central banks to stimulate the economy when traditional policy has become ineffective.
Commonly Used Financial Jargon
Navigating the seas of quantitative finance means being familiar with various jargon. Hereโs a brief overview:
- Alpha: A measure of the active return on an investment compared to a market index.
- Beta: Indicates the volatility of an asset or portfolio compared to the market as a whole.
- Liquidity: Refers to how easily assets can be converted into cash without affecting their market price.
- Volatility: A statistical measure of the dispersion of returns for a given security or market index.
Understanding these terms will provide a firm foundation as we progress further into the topic of quantitative methods.
Glossary of Investment Products
A comprehensive grasp of the different financial products available in the market is invaluable for investors. Each product serves a specific purpose and aids in fulfilling various investment strategies. Here are a few commonly used products:
- Stocks: Equity securities representing a claim on a company's assets and earnings.
- Bonds: Debt securities issued to raise capital, promising to pay interest at specified intervals.
- Mutual Funds: Investment vehicles that pool money from multiple investors to purchase a diversified portfolio of stocks and/or bonds.
- Exchange-Traded Funds (ETFs): Similar to mutual funds but traded on stock exchanges.
Each of these products comes with unique strategies:
- Growth Investing: Focuses on stocks expected to grow at an above-average rate compared to their industry.
- Value Investing: Involves picking stocks that appear to be undervalued in price.
- Income Investing: Investment strategy focused on generating regular income from investments, usually through dividends.
These products and strategies form the backbone of financial markets and understanding them can empower investors to make more calculated decisions.
In finance, knowledge is often the best investment.
As we move forward, itโs essential to keep these concepts at the forefront of your mind. They will not only aid in understanding quantitative methods more deeply but also enhance your overall investment strategy.
Foreword to Quantitative Methods in Finance
Quantitative methods have emerged as a cornerstone in the field of finance, reshaping how investors and institutions approach financial decision-making. In todayโs world, where data is abundant and market dynamics are increasingly complex, understanding these methods is not merely advantageous; itโs essential. Investors are seeking to adapt to rapidly changing environments and to maintain a competitive edge. With quantitative techniques, they analyze vast volumes of data with an unprecedented level of detail, yielding insights that once seemed elusive.
Definition and Significance
At its core, qualitative finance hinges on the application of statistical and mathematical models to understand and predict market behavior. This area is not reserved for the usual suspects of Wall Street; even small investors can leverage these methods to refine their strategies.
The significance of quantitative methods lies in its ability to streamline processes and reduce the uncertainty related to investment choices. Each model serves as a lens, providing clarity through which investors analyze risks and returns. Furthermore, this approach enables better portfolio optimization and enhances the assessment of asset performance over time.
"The ability to quantify risk allows investors to make informed decisions, transforming anecdotal evidence into actionable insights."
When investors embrace these methods, they trade in the guesswork of intuition for models grounded in data. This pivot towards quantification can lead to improved risk management practices and a more robust investment strategy, aligning closely with the principles of modern finance.
Historical Context
Delving into the historical context of quantitative methods unveils the evolution of financial thought. From the inception of portfolio theory in the mid-20th century to contemporary advancements in machine learning and AI, the landscape has changed tremendously.
The 1952 work of Harry Markowitz introduced the concept of efficient frontiers, compelling investors to consider risk and return in a systematic way. Fast forward to the 1970s, the Black-Scholes model transformed options pricing, allowing for better strategies to hedge against losses. Today, we find ourselves in an era where algorithms and automated trading dominate, reflecting a seamless integration of quantitative techniques across all levels of finance.
Incorporating these methodologies not only revolutionized individual trading but also influenced large institutional strategies, facilitating dynamic responses to market fluctuations. The journey showcases the growing recognition of analysis laden with data as a guiding force in financeโa necessity rather than a luxury.
Key Quantitative Techniques
Quantitative techniques serve as the backbone for several financial analyses and investment strategies within the finance sector. Understanding different methods of analysis is crucial as they provide the tools needed to interpret vast data sets and forecast market movements. When wielded correctly, these techniques can aid investors in making informed and strategic decisions, thus safeguarding assets and maximizing potential returns.
Statistical Analysis
Statistical analysis is at the heart of quantitative finance. It encompasses various methodologies for collecting, reviewing, and interpreting data. At its core, statistical analysis aids in understanding trends, making predictions, and assessing risk.
For instance, analysts might utilize descriptive statistics to summarize a range of financial datasets, representing figures such as mean, median, and standard deviation. These metrics offer a snapshot view, shaping strategies grounded in fundamental observations. Furthermore, inferential statistics allow investors to make generalizations about larger populations from sample data, giving them insight into potential market shifts or investment opportunities.
Important elements of statistical analysis include:
- Data Collection: Gathering reliable data is pivotal. Analysis is only as good as the data it uses.
- Hypothesis Testing: This process allows for the validation of assumptions regarding financial models. Itโs not just about the numbers but understanding their implications.
- Data Visualization: Using graphs and charts (like histograms and pie charts) can make analysis intuitive, promoting clearer communication of findings among stakeholders.
โStatistical attention is less about blinding operators with numbers and more about clarifying investment pathways through analytics.โ
Regression Analysis
Regression analysis further deepens the understanding of relationships between variables in finance, such as the relationship between a stockโs price and market indicators. This technique provides clarity on how dependent variables interact with independent variables over time, which can affirm or challenge existing beliefs about market functions.
For example, a financial analyst may use regression to evaluate how interest rates affect stock prices. By stating that for each percentage point increase in interest rates, a certain percentage drop may occur in stock prices, investors can adjust their portfolios accordingly.
Key aspects of regression analysis include:
- Simple Regression: Examines the relationship between two variables. Think of predicting sales based on advertising spend.
- Multiple Regression: Involves more than one independent variable, capturing the nuanced interactions influencing financial outcomes.
- R-squared Value: This statistic indicates the proportion of variance for the dependent variable explained by the independent variables. The higher this value, the stronger the modelโs explanatory power.
Regression analysis is invaluable for investors striving to forecast trends accurately and make informed decisions based on historical data.
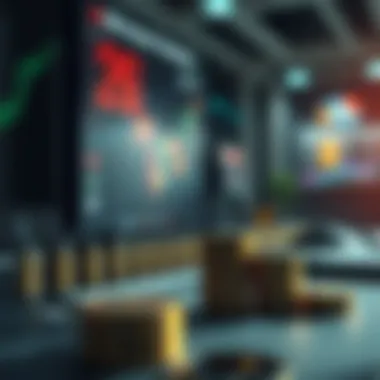
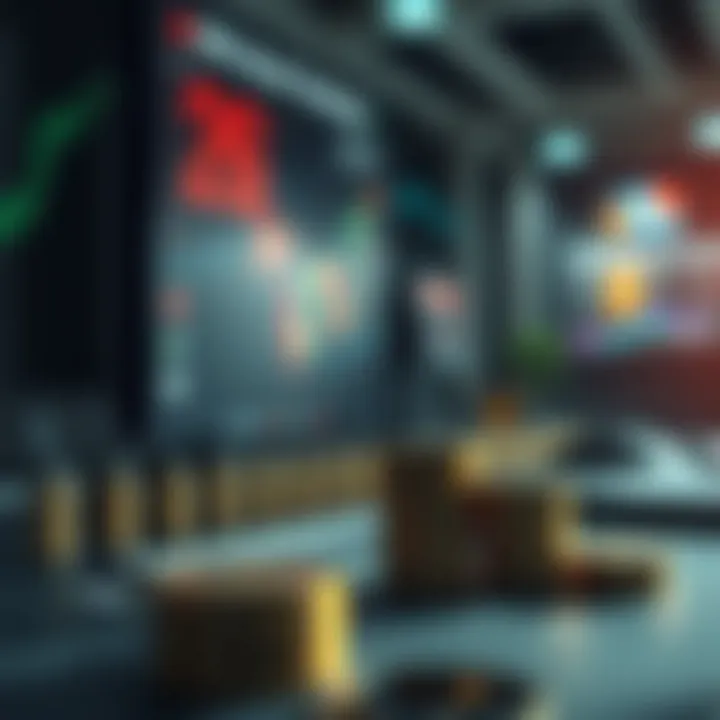
Time Series Analysis
Time series analysis traces data points collected or recorded at specific intervals, mainly focusing on trends over time. This technique is essential in finance since it helps investors analyze stock price movements, economic indicators, and other financial metrics over continuous time frames.
For instance, through time series analysis, an analyst can identify seasonal variations in stock prices by examining historical price movements over months and years. Understanding these patterns assists investors in predicting potential price fluctuations and adjusting their trading strategies accordingly.
Significant features of time series analysis include:
- Trend Analysis: Reveals underlying trends or directions over time. Investors may track upward or downward trends to guide their buying or selling actions.
- Seasonal Decomposition: Breaking down the time series into seasonal factors helps investors distinguish cyclical patterns from irregular movements, leading to better predictions.
- ARIMA Models: Autoregressive Integrated Moving Average (ARIMA) is a tool used in time series forecasting that combines autoregressive and moving average components. Itโs particularly valuable for its predictive capabilities.
By mastering time series analysis, investors are better equipped to navigate the complexities of markets and economic conditions, helping them stay one step ahead.
Financial Modelling
Financial modelling represents a pivotal concept within the landscape of quantitative finance, functioning as a blueprint that captures the varying dynamics of financial conditions and forecasts future performance. These models blend theoretical frameworks with empirical data, allowing analysts and investors to make informed decisions based on quantitative insights.
In todayโs fast-paced financial environment, the significance of robust financial models cannot be overstated. They serve multiple purposes, including valuation of assets, assessment of financial risks, and the simulation of various market scenarios. By clarifying complex financial variables through structured simulations and outputs, these models enhance understanding, guide strategic planning, and help better manage investment portfolios.
In developing financial models, several key considerations arise:
- Assumptions: Every model hinges on assumptions about market behavior, interest rates, and economic indicators. These assumptions must align closely with real-world conditions to ensure the model's accuracy.
- ** Data Integrity**: The old adage "garbage in, garbage out" rings true in finance too. Employing accurate and current data is essential for reliable model outputs.
- Flexibility and Updates: Financial models should not be static. They require regular updates based on new data and changing market conditions to remain relevant and useful.
A sound financial model is not just a mathematical exercise; it reflects an understanding of underlying economic realities and a projection of future states influenced by those realities.
This section dives into the various types of financial models and how they are implemented in a practical context.
Types of Financial Models
Financial models can vary greatly, each serving its specific function and tailored to unique situations. Here are several commonly used types:
- Discounted Cash Flow (DCF) Models: These models estimate an investment's value based on its expected future cash flows, distinguished by their present value calculations. They often focus on concepts like free cash flow and terminal value, making it essential for valuing a variety of assets.
- Comparative Company Analysis Models: This technique involves valuing a company based on how similar firms are priced in the market. By assessing multiples such as P/E ratios, analysts can gauge relative value.
- Risk Assessment Models: Used primarily in risk management, these models help identify potential financial losses and assign probabilities to various financial scenarios.
- Portfolio Models: These frameworks assist investors in determining ideal asset allocations by assessing risk versus return trade-offs among various investments.
- Option Pricing Models: Models like the Black-Scholes formula calculate the theoretical value of options based on variables such as the underlying asset price, strike price, volatility, and time to expiration.
Adopting the correct model largely depends on an individualโs objective, whether for investment strategies, valuation, or risk assessment, highlighting the diverse applicability of financial modelling in finance.
Model Validation and Testing
Once a financial model is established, ensuring its validity becomes paramount. Model validation refers to the process of evaluating a financial modelโs ability to accurately predict outcomes based on historical data. The distinction between a theoretical model and one that works in practice can be vast.
Several methods can be employed for validation:
- Backtesting: This technique involves applying the model to historical data to assess its predictive capability. If the model performs well with past data, it instills confidence in its ability to forecast future conditions.
- Cross-Validation: This approach divides available data into multiple subsets, using some for training the model while others are reserved for testing. This helps ensure robustness in different market scenarios.
- Stress Testing: This process puts the model under extreme conditions to evaluate how it stands up to significant market shifts, such as sudden economic downturns or rapid interest rate changes.
- Peer Review: Involving colleagues or external experts in model evaluation can provide valuable insights and identify potential flaws before the model is put into action.
Risk Management Techniques
Risk management is a crucial pillar in the realm of finance. It encompasses a myriad of strategies and methodologies designed to identify, assess, and mitigate potential financial risks. Given the inherent uncertainties in financial markets, effective risk management not only safeguards investments but also enhances decision-making processes. In this section, we will delve deeper into key techniques, focusing on Value at Risk (VaR) and Stress Testing.
Value at Risk (VaR)
Value at Risk (VaR) is perhaps one of the most well-recognized risk management methodologies used by financial professionals. At its core, VaR quantifies the maximum expected loss on an investment over a given time period at a specified confidence level. This means it offers insights into how much of a financial hit an investor might take within a particular time frame under normal market conditions.
For instance, if a trader reports a VaR of $1 million at a 95% confidence level over one day, it implies thereโs a 5% chance that losses could exceed this amount on any given day. The beauty of VaR lies in its simplicity and its ability to facilitate comprehensive risk assessment across varied portfolios.
- Key Benefits of VaR:
- Provides a clear metric to evaluate risk exposure.
- Facilitates comparisons between different investment options.
- Aids in regulatory compliance, as many financial institutions are required to adhere to certain risk measures.
However, it is crucial to bear in mind several limitations associated with VaR. It does not capture extreme market movements very well, which can lead to underestimating risk during volatile times. The reliance on historical data also brings into question the accuracy of models in forecasting future losses.
"While VaR provides a basis for risk analysis, itโs paramount for investors and managers to complement it with other risk metrics to form a more holistic understanding of potential threats."
Stress Testing
Stress testing is another robust technique employed in the financial sector aimed at assessing how certain stress conditions could affect a financial institutionโs stability. This involves simulating various hypothetical scenariosโsuch as economic downturns, market shocks, or geopolitical eventsโto analyze how these events would impact portfolio returns.
Unlike VaR, stress testing goes beyond looking at statistical probabilities to simulate extreme market conditions. This can involve changes in interest rates, drastic drops in asset prices, or rapid shifts in currency values. It provides insights into potential vulnerabilities in investments and helps institutions prepare contingency plans.
- Key Features of Stress Testing:
- Identifies potential weaknesses in the financial structure.
- Encourages proactive risk management and strategic planning.
- Enhances regulatory compliance by demonstrating robustness in the face of adverse scenarios.
Stress tests can be tailored to suit individual portfolios, allowing investment managers to gauge their resilience under various hypothetical adverse conditions, ultimately leading to more informed and resilient decision-making.
Both VaR and stress testing are vital components of risk management techniques that can greatly influence financial strategies. By integrating these methodologies, investors and institutions can bolster their capability to weather market uncertainties and enhance their overall financial health.
Algorithmic Trading
Algorithmic trading has revolutionized the financial landscape, employing mathematical models to execute trades at speeds and volumes unmatched by human traders. The significance lies in its ability to capitalize on market inefficiencies, ensuring data-driven decision-making that can enhance profitability while mitigating risks. By analyzing price trends and patterns, algorithms can process vast amounts of informationโfar beyond human capacityโthus enabling investors to seize trade opportunities in real-time.
Concept and Implementation
The concept of algorithmic trading revolves around algorithms, which are sets of rules or instructions designed to perform tasks involving complex decision-making. These algorithms evaluate factors like order book data, price movements, and historical trends to execute trades. To implement algorithmic trading successfully, one must begin with a well-defined strategy. This could be as simple as arbitrageโprofiting from price differences across marketsโor as complex as high-frequency trading, where trades are executed within milliseconds.
Key steps in implementation include:
- Backtesting: This is where the algorithmโs effectiveness is assessed using historical data. The aim is to determine if the strategy would have been profitable in the past.
- Execution: Once the algorithm passes backtesting, itโs deployed in live markets with real orders. Execution speed is critical, and thus, the infrastructure must support latency-sensitive trading.
- Monitoring: Continuous evaluation is necessary to ensure the algorithm performs as expected in different market conditions. Adjustments may be necessary based on changing market dynamics.
The success of algorithmic trading often hinges on the intricacies of implementation rather than merely the underlying strategy. Investors can harness sophisticated programming languages such as Python or R to develop these algorithms, making the technical skills quite essential.
Performance Evaluation
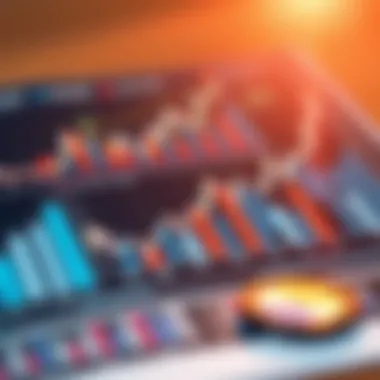
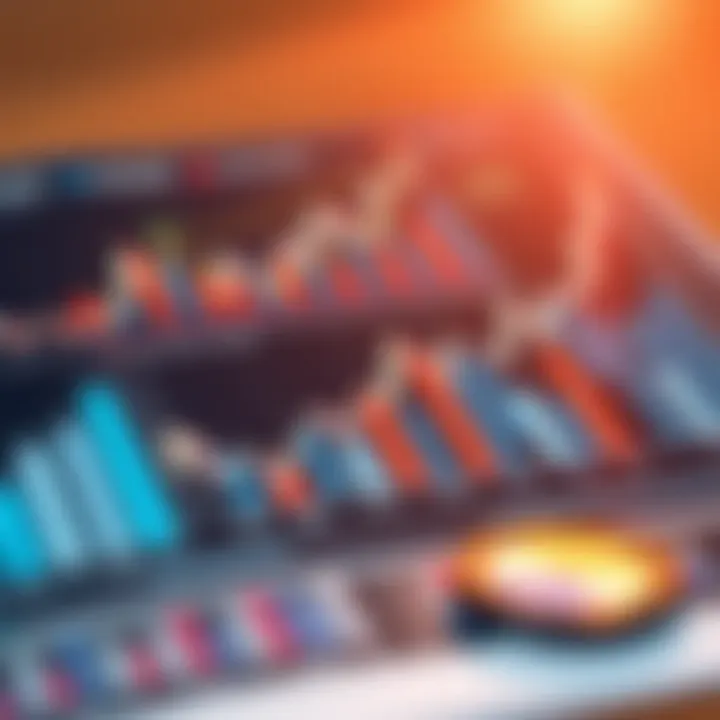
Evaluating the performance of algorithms involves assessing various metrics, providing a holistic view of their effectiveness. A few critical metrics include:
- Sharpe Ratio: Measures return per unit of risk; higher is better.
- Maximum Drawdown: Indicates the maximum observed loss from a peak to a trough; important for risk assessment.
- Win Rate: The percentage of profitable trades; though not the sole indicator of success, a higher rate generally points to an effective algorithm.
Furthermore, analyzing factors such as execution quality, slippage, and transaction costs forms a part of the evaluation process. It's essential to compare the results against a relevant benchmarkโlike market indicesโto gauge relative performance.
"In the world of finance, where milliseconds can mean the difference between profit and loss, the role of algorithmic trading cannot be overstated."
In summary, algorithmic trading stands as a powerful tool in the arsenal of modern finance, enabling systematic trading strategies that can adapt swiftly to market movements. Mastering the implementation and evaluation of such algorithms is crucial for any serious investor or trading professional.
Data Analysis Tools
In the realm of quantitative finance, the ability to effectively analyze data is tantamount to success. Data analysis tools play a crucial role in enabling financial enthusiasts and individual investors to make informed decisions. The right tools allow for the transformation of raw data into actionable insights, which can ultimately lead to better investment strategies and risk management practices.
Statistical Software
Statistical software packages are indispensable in quantitative finance. Tools like R, SAS, and Stata provide robust environments for data manipulation and statistical modeling. They help professionals to run complex analyses, from basic descriptive statistics to intricate multivariate tests. For instance, R provides an open-source platform, which comes with extensive libraries tailored for financial analysis. This means users can easily perform sophisticated tasks like time series forecasting or regression modeling without needing to write from scratch.
Moreover, statistical software facilitates the replication of models, allowing users to validate their results and ensure reliability. This is particularly vital in finance, where decision-making is heavily reliant on accurate forecasts and analyses. Hereโs a quick rundown of some noteworthy statistical tools:
- R: Great for statistical analysis and plotting.
- SAS: Known for data management and advanced analytics.
- Stata: User-friendly interface for data manipulation and statistical techniques.
In the world of finance, "data is the new oil"โitโs all about how you refine it that matters. With statistical software, data becomes a valuable resource that fuels strategic financial initiatives.
Programming Languages
Programming languages form the backbone of sophisticated quantitative methods. Languages such as Python, C++, and MATLAB are prevalent in the finance sector due to their capabilities in handling large datasets and performing computationally intensive tasks. For example, Python is renowned for its simplicity and readability, making it an excellent choice for those who might not have a strong programming background.
Python also boasts libraries like Pandas for data manipulation and NumPy for numerical analysis, which can significantly streamline processes. This efficiency allows analysts to focus on strategic decisions rather than getting bogged down by the mechanics of data processing. The advantages of programming languages include:
- Flexibility: Customize analyses to meet specific needs.
- Speed: Efficient computation, especially with large volumes of data.
- Automation: Ability to automate repetitive tasks, freeing up time for strategic thinking.
Programming in the context of quantitative finance doesnโt have to be intimidating. Many free resources and communities, like Stack Overflow and Kaggle, provide opportunities for new learners and seasoned experts to exchange knowledge.
Key Takeaway: Mastering data analysis toolsโboth statistical software and programming languagesโempowers finance professionals to enhance their analytical capabilities, ultimately leading to more informed investment strategies and improved risk management.
Applications in Investment Strategies
In the domain of finance, strategies are only as good as the data and methodologies that back them. Quantitative methods have become an essential pillar in shaping investment strategies that are responsive to market changes and investor goals. Understanding quantitative applications in investment helps individuals and institutions alike harness the power of data for strategic advantages. This section digs deep into how quantitative analysis is applied in investment portfolios, emphasizing its benefits and considerations that investors must keep in mind.
Portfolio Optimization
Portfolio optimization refers to the process of selecting the best mix of assets to maximize returns while minimizing risk. This is where quantitative methods come into play, using mathematical frameworks and statistical tools to inform decision-making. Investors today seek to strike a balance between risk and return and employing quantitative techniques allows for a more sophisticated approach than traditional methods.
One popular quantitative method is the Mean-Variance Optimization. This framework calculates the expected return of an asset, taking into account the variance and covariances among assets in a portfolio. By simulating various scenarios and asset distributions, investors can identify optimal allocations that deliver the highest return for a given level of risk.
Key aspects to consider when optimizing a portfolio include:
- Risk Tolerance: Investors need to understand their risk profile which affects asset weights in the portfolio.
- Diversification: Spreading investments across various asset classes can reduce unsystematic risk.
- Current Market Conditions: Market volatility may necessitate adjustments in asset allocation to align with changing risk/reward dynamics.
"Investing is like a minefield; one misstep can change everything entirely." โ Unknown
Asset Allocation Techniques
Asset allocation is more than just picking stocks; it's a comprehensive strategy that encompasses a blend of different asset classes to achieve specific financial goals. Quantitative analysis enhances the asset allocation process by employing statistical models that optimize investment distribution based on historical and predicted market behaviors.
Common types of asset allocation techniques include:
- Strategic Asset Allocation: It involves setting long-term targets for different asset classes, adjusting periodically based on market conditions.
- Tactical Asset Allocation: This is a more flexible approach where investors adjust their asset mix based on short-term economic outlooks.
- Dynamic Asset Allocation: This method actively reallocates investments as markets change, reacting quickly to fluctuations in real time.
Incorporating quantitative techniques in asset allocation can help identify trends and correlations that are not immediately apparent to the naked eye. For instance, using machine learning algorithms enhances forecasting power, enabling tailored approaches based on complex data sets. Employing these methods provides investors with a competitive edge that can yield significant returns in uncertain markets, ensuring that they keep their finger on the pulse of the ever-changing financial landscape.
Quantitative Risk Assessment
Quantitative risk assessment plays a pivotal role in finance, serving as the backbone for sound investment strategies. It encompasses a range of methodologies that allow financial professionals to gauge potential losses and determine the probability of adverse events affecting their portfolios. In an age where financial markets can shift in the blink of an eye, understanding the intricacies of quantitative risk assessment is no longer optional but essential.
Quantitative risk assessment involves applying mathematical models to evaluate and predict risk factors. This helps investors make data-driven decisions rather than relying purely on gut feelings or traditional economic theories. By quantifying risks, firms can allocate resources more efficiently, identify vulnerabilities in their portfolios, and respond proactively to market volatility. Thus, this approach not only safeguards against unexpected downturns but also positions investors to capitalize on emerging opportunities.
Key Metrics and Indicators
Understanding risk through the lens of quantitative assessment relies heavily on specific metrics and indicators. Some of these key metrics include:
- Value at Risk (VaR): This metric estimates the maximum potential loss over a specified period for a given confidence interval. For instance, if a portfolio has a one-day VaR of $1 million at a 95% confidence level, it suggests that there is a 5% chance that losses could exceed $1 million in a single day.
- Standard Deviation: This statistical measure quantifies the amount of variation in a set of data points. In finance, a higher standard deviation indicates greater volatility, suggesting that the asset's price can fluctuate substantially.
- Beta: This measures a stock's volatility relative to the overall market. A beta greater than one indicates that the asset is more volatile than the market, while a beta less than one shows it is less volatile.
- Sharpe Ratio: This ratio indicates how well an investment compensates for the risk taken. A higher Sharpe ratio suggests a more attractive risk-adjusted return.
These metrics, when correctly interpreted, provide crucial insights into the potential risks associated with investments, enabling stakeholders to make informed decisions in the complex realm of finance.
Limitations of Quantitative Analysis
While the benefits of quantitative risk assessment are substantial, itโs crucial to recognize its limitations. These methods are not infallible and can falter for several reasons:
- Model Risk: Every model is a simplified version of reality. If the assumptions underlying a model prove to be incorrect, the outcomes it predicts can lead to misguided investment strategies.
- Data Quality: The reliance on historical data can pose challenges. Markets are dynamic, and relying solely on past performance may not adequately predict future risks. Poor data quality can exacerbate this issue, leading to inaccurate risk assessments.
- Behavioral Factors: Human emotions and irrational behaviors often play a significant role in market movements. Quantitative models tend to overlook these psychological elements, which can skew their effectiveness.
- Overfitting: Sometimes, models are too tailored to historical data, which severely limits their applicability in changing market conditions. This overfitting can result in poor performance when applied to future scenarios.
In summary, while quantitative risk assessment is fundamental for effective financial decision-making, it is essential to approach it with caution. Investors should combine these quantitative tools with qualitative insights for a holistic view of risk. This balanced approach can ultimately enhance the robustness of financial strategies and avoid pitfalls inherent in relying solely on numerical models.
"Quantitative methods are valuable tools in finance, but like all tools, their effectiveness depends on how well they are wielded."
For further reading on financial metrics and risk assessment methodologies, check out resources available on en.wikipedia.org and investopedia.com.
The Future of Quantitative Finance
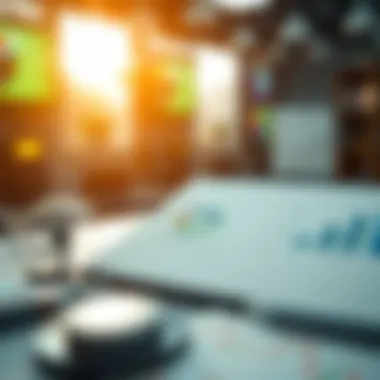
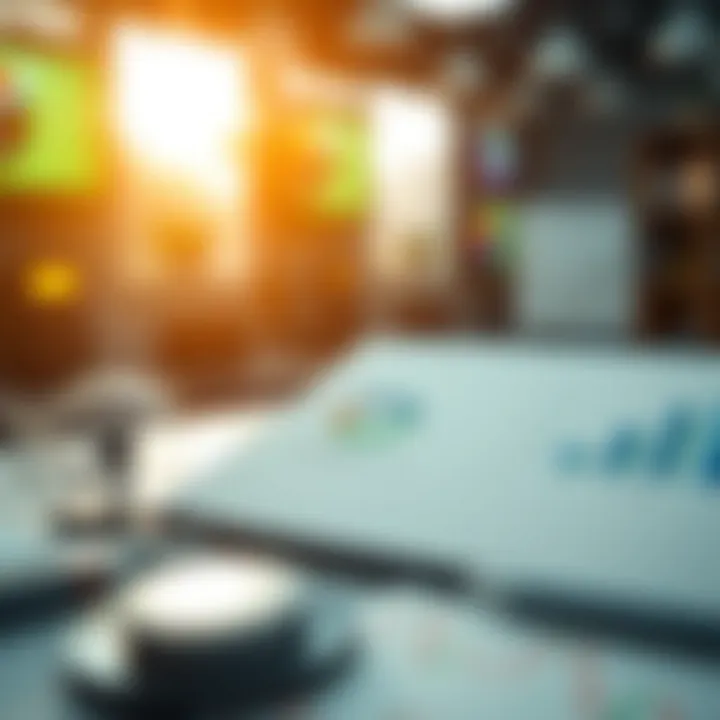
As the financial landscape continuously transforms, the future of quantitative finance is poised for exciting advancements. This section highlights emerging trends and the integration of cutting-edge technologies, particularly artificial intelligence, which are shaping the fundamentals of this field. Understanding these facets is crucial for financial professionals seeking an edge in a competitive environment.
Emerging Trends
New developments are sprouting up like weeds, as technology reshapes the financial world. Among the emerging trends, a few stand out as particularly significant:
- Big Data Utilization: The vast amounts of data now available are like a gold mine for quantitative analysts. By leveraging big data analytics, they can uncover patterns and insights hidden beneath the surface, leading to more informed decision-making and strategy formulation.
- Machine Learning: This method is becoming the bread and butter for many market participants. Algorithms can learn from data, improving their predictive capabilities over time. Financial professionals are increasingly implementing these techniques to gain an upper hand in algorithmic trading and risk assessment.
- Quantum Computing: As quantum capabilities become more accessible, financial models that once took hours to compute will be processed in mere seconds. This transformation holds great potential for optimizing complex financial problems, enabling unprecedented levels of efficiency.
"The advent of quantum computing could revolutionize how we approach financial modeling and risk analysis, making previously impractical calculations realizable."
- Behavioral Finance Insights: Understanding investor psychology is gaining traction. Incorporating behavioral finance models alongside traditional quantitative methods could provide a more holistic view of market dynamics. Acknowledging the human element in finance may lead to superior investment strategies.
Integration of Artificial Intelligence
Artificial intelligence is not just a buzzword; itโs becoming integral to the future of quantitative finance. Firms are increasingly employing AI for several key purposes:
- Automated Trading Systems: AI-driven algorithms can analyze market conditions and execute trades in fractions of a second. This speed helps exploit fleeting opportunities that may be invisible to human traders.
- Risk Management Enhancements: By employing machine learning models, organizations can identify and mitigate risks more proactively. AI can analyze vast datasets for emerging threats, allowing for prompt action before issues escalate.
- Personalized Financial Services: Clients appreciate tailored investment solutions. AI can assess individual risk appetites, investment wishes, and market trends to construct customized portfolios.
- Sentiment Analysis: Understanding market sentiment through AI can reveal how news and events influence stock prices. Analyzing social media, news articles, and market chatter helps refine predictions and develop adaptable trading strategies.
The integration of artificial intelligence into finance doesnโt come without challenges. Data biases, ethical considerations, and the need for transparency in algorithms are ongoing discussions that financial leaders must navigate.
As the fields of machine learning and AI expand, professionals in quantitative finance must adapt. Staying abreast of these advancements will be essential for those aiming to thrive in an ever-evolving industry.
Challenges in Quantitative Finance
In the realm of quantitative finance, challenges abound. Understanding these hurdles is pivotal for anyone virbantly interacting with or studying this field. The importance of grasping these issues cannot be understated; after all, they dictate the nuances of investment outcomes, the efficacy of risk strategies, and the overall robustness of financial models. More than mere academic exercises, addressing these challenges can lead to more effective decision-making and strategy implementation.
Data Quality Issues
Data quality issues stand as a significant hurdle within quantitative finance. The raw numbers that analysts, traders, and researchers rely on can often be less than stellar. Errors in data collection, processing, or even parsing can skew results dramatically. This isn't merely a theoretical concern; poor data quality can lead to suboptimal investment strategies, which might cost investors dearly. If financial modeling is built on shaky foundations, the consequences can cascade into poor forecasting, misplaced risk assessments, and ultimately, financial losses.
Consider the case where historical price data for a stock is manipulated or incorrectly reported. An analyst utilizing this flawed data to define trends may draw entirely incorrect conclusionsโbelieving a stock is on the rise while it is heading south.
Here are some critical aspects to consider regarding data quality:
- Source Reliability: Only rely on data from trusted sources to minimize the likelihood of errors.
- Data Validation: Implement robust validation processes to check for inconsistencies and inaccuracies in datasets.
- Regular Updates: Ensure that data sets are kept up to date, as outdated information can mislead investment decisions.
"Good decisions come from experience, and experience comes from bad decisions."- Mark Twain
Model Risk
Model risk is another challenge that cannot be overlooked. Simply put, it's the risk of a model providing inaccurate predictions or outcomes. This is often due to flawed assumptions or oversimplifications in the model's design. For an industry that relies so heavily on models for forecasting, pricing, and risk assessment, this can be an eye-opening concern.
Financial models often rest on a series of assumptions which, if proven wrong, can have dire implications. For example, a valuation model might assume a constant growth rate, but market conditions can be unpredictable and volatile. If those assumptions do not hold true, the modelโs output loses integrity almost instantly.
Here's what to bear in mind when thinking about model risk:
- Sensitivity Analysis: Conduct sensitivity tests to see how changes in inputs affect outputs, helping to identify potential weaknesses.
- Periodic Re-Evaluation: Reassess models regularly to ensure they adapt with changing market environments or data.
- Diverse Approaches: Utilize multiple modeling techniques to provide a more rounded view and mitigate reliance on a single structure.
Overall, navigating through the challenges of data quality and model risk is essential for anyone engaged in quantitative finance. Acknowledging these hurdles not only allows for rectifying weaknesses in financial modeling but fosters better decision-making in investment and financial strategy.
Case Studies in Quantitative Finance
Case studies in quantitative finance play a vital role in demonstrating how theoretical concepts can translate into real-world applications. They provide an effective lens through which we can assess the success or failure of quantitative methods in action. From successful implementations that lead to substantial financial gains to cautionary tales where miscalculations or misapplications resulted in significant losses, these narratives help both seasoned and novice investors understand the practical aspects of quantitative finance.
By examining specific instances, we can glean insights into the nuances of quantitative techniques, offering a richer understanding than theoretical discussions alone.
Successful Implementations
Successful implementations of quantitative methods often showcase the power of data-driven decision-making in finance. For instance, consider the case of Renaissance Technologies, a quantitative hedge fund known for its systematic trading strategies. Their success is largely attributable to statistical arbitrage, a technique that exploits pricing inefficiencies in the market by identifying patterns and trends within massive datasets.
In this case, the fund captured numerous opportunities by leveraging advanced algorithms that analyze historical data. They utilized robust statistical methods to identify correlations across various assets, resulting in a consistently profitable trading strategy over the years. The case of Renaissance Technologies is proof that when quantitative methods are applied correctly, they can generate remarkable outcomes.
Another noteworthy example is the application of machine learning in credit scoring models, primarily used by companies like ZestFinance. This company enhanced traditional credit assessment by integrating additional data points, such as payment histories from alternative sources. These models yielded improved predictive power, allowing lenders to make more informed decisions and decrease default rates. The result? Lower risk and higher profitability for lenders.
"Quantitative finance isn't just about algorithms; itโs about turning data into decisions that drive profit."
Failures and Lessons Learned
While success stories abound, the quantitative finance landscape is not without its failures. The "Long-Term Capital Management" (LTCM) catastrophe in the late 1990s stands as a classic example. The hedge fund employed sophisticated mathematical models based on historical performance. Initially, it yielded impressive returns, but when confronted with a market crisis, LTCMโs models failed to predict extreme events, leading to disastrous financial outcomes and ultimately requiring a bailout.
This incident highlighted a critical lesson: over-reliance on models without considering macroeconomic factors or real market behaviors can lead to perilous situations.
In addition, the failure of ledgers during the 2008 financial crisis, especially in mortgage-backed securities, illustrated how algorithmic trading could exacerbate market downturns. Many quant-driven strategies faltered during this time as the models underestimated the risks associated with bundled mortgages.
These cautionary tales reinforce the importance of integrating qualitative insights with quantitative analysis. Itโs vital to understand that models are approximations of reality and can miss unforeseen scenarios.
By analyzing failures alongside their successes, financial professionals can cultivate a sense of humility regarding their methodologies and build more adaptive and resilient strategies.
Culmination
The conclusion of this exploration into quantitative methods in finance serves as the capstone that brings together the key threads woven throughout the article. Understanding how these methods function is critical for investors and financial analysts alike, providing a structured framework for decision-making in an ever-evolving financial landscape.
Summary of Insights
In reviewing the material discussed, it becomes clear that quantitative methods are not merely tools for number crunching; they are crucial to formulating strategies that are data-driven. The techniques such as statistical analysis, regression models, and time series forecasting offer various lenses through which market behaviors can be scrutinized.
- Statistical Analysis helps dissect market data to identify trends and anomalies.
- Regression Techniques allow for the determination of relationships between variables, enhancing predictive accuracy.
- Time Series Analysis assists in forecasting future price movements based on historical data patterns, which is pivotal for proactive investment strategies.
Together, these techniques empower investors to make informed choices based on empirical evidence rather than instincts. The emphasis on rigorous modeling and robust testing ensures that financial predictions are not shot in the dark but are anchored in reality.
Future Implications for Investors
Looking ahead, the implications of embracing quantitative methods continue to expand. With the rise of big data and advancements in machine learning, the integration of these technologies into quantitative finance practices is no longer a distant possibility but an ongoing trend. Investors who recognize and adapt to these changes are likely to gain a competitive edge.
Key considerations for the future include:
- Artificial Intelligence integration can optimize trading algorithms, making them more responsive and intelligent.
- Data Visualization Technologies will help investors better comprehend complex datasets and reveal insights more clearly.
- Risk Management Tools that leverage quantitative models can provide unprecedented foresight, safeguarding portfolios against market volatility.